For professional service sales teams, complex service quotes often determine whether new deals are won or lost. Getting these quotes right requires striking a balance between precision, speed, and insight into project requirements. One way to achieve better accuracy is to incorporate historical data into your quoting process. By examining past projects—both successes and shortfalls—you can refine estimates, mitigate risks, and boost client satisfaction. To learn more about related strategies, you might explore our guide on how to automate complex service scoping and quoting for professional sales teams.
Why Historical Data Matters for Complex Service Quotes
Historical data provides a factual baseline for understanding the real-world costs, timelines, and resources tied to complex service work. Whether you deliver consulting, IT services, or other professional engagements, every past project holds lessons waiting to be uncovered. By analyzing what went well—and what did not—you gain context for upcoming quotes that pure guesswork can’t match.
A report by the Project Management Institute found that only 57% of projects are completed within their original budgets (1). Such overruns often stem from inaccurate estimating. When you tap into historical data, you can spot recurring pitfalls, identify resource bottlenecks, and better determine how much labor or time a new project will actually require.
Collecting and Organizing Past Project Data
Gathering robust information from completed projects is the first step. Start by:
• Capturing the Full Cost Picture: Log expenses related to labor hours, subcontractors, equipment, licenses, and anything else that impacted total spend.
• Building a Centralized Repository: Store project details in one place—whether that’s a dedicated analytics platform or a single data warehouse—so they’re easy to find and compare.
• Standardizing Categories: Use consistent labels for project types, skill levels, or complexity tiers (e.g., “moderate” vs. “high complexity”). This consistency streamlines comparisons later.
• Tagging Risks and Outcomes: Note significant events such as scope changes, delays, or client-driven modifications. These factors skew your data if not documented.
Once your data is consistent, you can generate more accurate estimates. Patterns hidden in large volumes of information become easier to recognize, enabling you to anticipate factors like project duration or budget variations.
Techniques for Analyzing Historical Data
Once historical data is compiled and organized, a variety of techniques can tease out meaningful insights:
• Trend Analysis: Examine how costs and timelines shifted across similar projects over multiple months or years. Recurring surges or dips may point to specific times when you need to allocate extra resources.
• Pattern Recognition: Identify repeated relationships between project attributes and outcomes. For instance, you might notice that short timelines often result in overtime expenses.
• Predictive Modeling: Use analytics software or machine learning tools to build forecasts based on the patterns in your existing data. These models grow more accurate over time.
• Comparative Assessments: Compare quotes for similar projects that had different outcomes. Discrepancies might reveal opportunities for reworking an estimation approach.
A study by McKinsey & Company found that data-driven organizations are 23 times more likely to acquire new customers than those that rely mainly on intuition (2). For service providers, applying the same data-driven mindset to pricing and quoting can improve competitiveness and build client confidence.
Benchmarking Against Industry Standards
While internal data is vital, external benchmarks give you an objective point of reference. Benchmarking involves comparing your quoting practices and resource allocations with industry norms:
• Identify Metrics: Understand which metrics matter for your niche. Perhaps it’s the average hourly rate, average project duration, or margin of error in quotes.
• Gather External Data: Look at published reports, associations within your industry, or even aggregated data from tool providers.
• Understand Your Position: If your quotes run significantly higher or lower than industry averages, investigate why. Maybe your service level justifies a higher price, or maybe your cost structure is inflated.
• Update Regularly: Because market conditions change, keep an eye on new industry data to remain competitive.
By weaving in external benchmarks with historical internal data, you get a more balanced view of what constitutes a realistic quote for your market sector.
Risk Assessment and Contingency Planning
Many factors can disrupt even the most carefully crafted quote: shifting client requirements, technical hiccups, supply chain disruptions, or regulatory changes. Historical data shines a light on which risks appear most often and how severe their impact tends to be. Use this intelligence to:
• Assign Risk Scores: Tag certain project features (e.g., reliance on a single vendor) with a risk level based on past outcomes.
• Build Contingencies: Set aside extra time or budget for projects with elevated risk.
• Communicate Early: Let stakeholders know how you arrived at your risk assessment. Transparency about potential roadblocks can foster trust.
Risk-based buffer strategies can prevent cost overruns and missed deadlines. Rather than undermining profitability, they often strengthen margins by reducing unplanned expenditures.
Tools and Platforms for Data-Driven Quoting
Today’s market offers many tools to make data-driven quoting more accessible. Options range from simple spreadsheet templates to advanced analytics suites. Some professional service providers also adopt specialized solutions such as services CPQ (Configure, Price, Quote) platforms. If you’re curious about CPQ’s role in elevating quote accuracy, explore our overview of what is services CPQ and why it matters for professional services and sales teams.
In addition to CPQ, consider:
• Business Intelligence (BI) Platforms: Tools like Power BI or Tableau can quickly visualize patterns in historical data.
• CRM Integration: Sync quoting systems with your CRM to capture leads, client histories, and past quotes in one place.
• AI-Driven Estimation: Machine learning algorithms can churn through thousands of data points to generate refined quotes, factoring in correlations that manual analysis may miss.
The key is to find a solution that fits your organizational workflow, so your sales and service teams can move faster without sacrificing precision.
Common Pitfalls to Avoid
Even with robust historical data, mistakes can creep into the quoting process. Watch out for these missteps:
-
Overlooking Outliers
Unique projects might distort an average. Instead of lumping them into broad categories, treat outliers separately or document their anomalies. -
Failing to Update Data
If you only rely on data from a few years ago, you might miss market shifts or new cost structures. Keep collecting and refreshing your dataset. -
Relying on Data Alone
While quantitative insights are essential, remember to consult experts or past project leads for qualitative context. Data shows the “what,” but team experience often reveals the “why.”
-
Omitting Risk Buffers
Even a data-driven quote can falter if risk factors go unmanaged. Historical data should inform your contingency plans, not make them optional. -
Skipping Post-Project Reviews
Incorporate lessons from each project back into your data repository. Building a feedback loop ensures continuous improvement in your quoting method.
Final Insights
Complex service quotes are more than just numbers on a page—they’re an expression of your expertise, reliability, and willingness to deliver on promises. Historical data offers a powerful way to refine those quotes. By studying real project outcomes, teasing out trends, and integrating benchmarks, you position your team to produce estimates that resonate with clients and maintain healthy profit margins.
If inaccuracies have hurt your business in the past, take the next step toward stronger estimates by reviewing why inaccurate scopes hurt your business and how to fix them. When you combine data-driven insights with thoughtful risk planning and modern quoting tools, the result can be a process that not only wins deals but also fosters long-term trust.
References
- Project Management Institute. (2022). “Pulse of the Profession.” Available at: https://www.pmi.org/learning/thought-leadership/pulse
- McKinsey & Company. (2019). “Analytics Comes of Age.” Available at: https://www.mckinsey.com/business-functions/mckinsey-analytics
Shachar Kaufman
Category
Related Posts
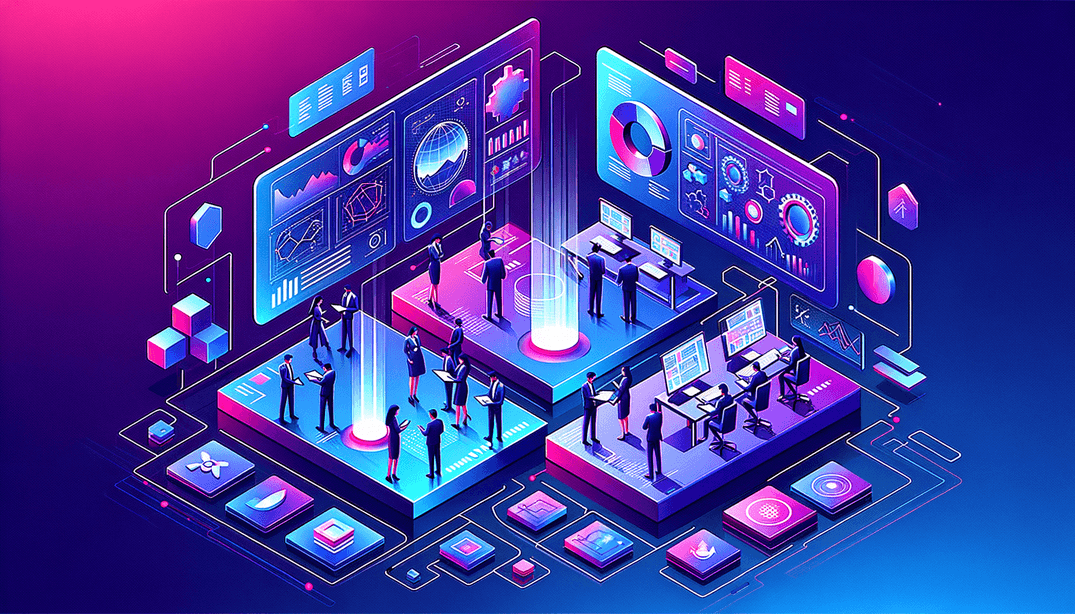
How to Streamline Pre-Sales Workflows for Better Scoping and Quoting
March 2025 | 83 min read
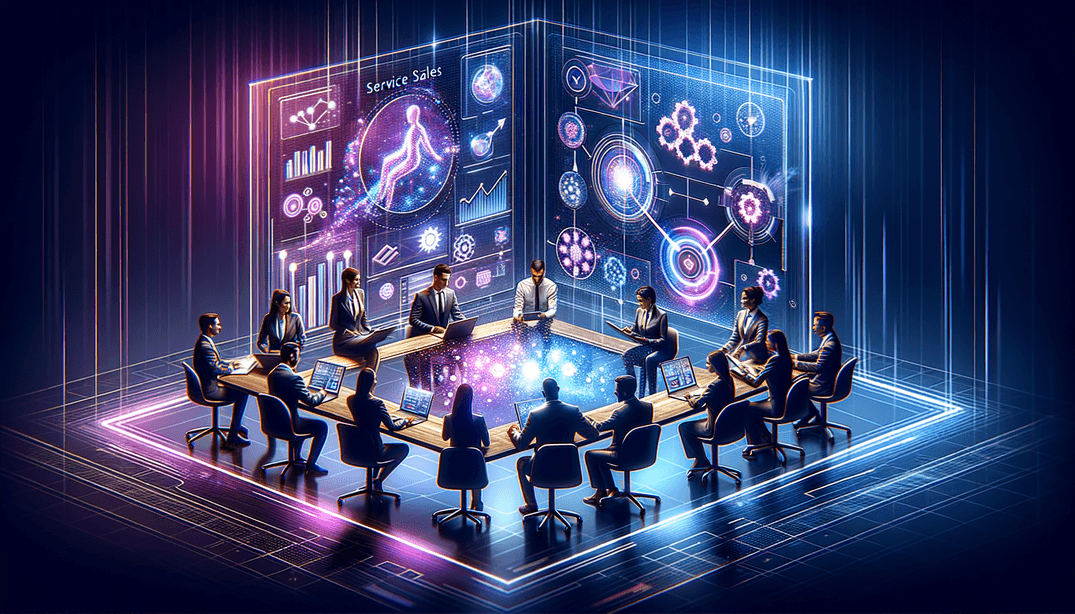
Why Service Sales Teams Struggle With Scoping and How Automation Can Help
March 2025 | 76 min read
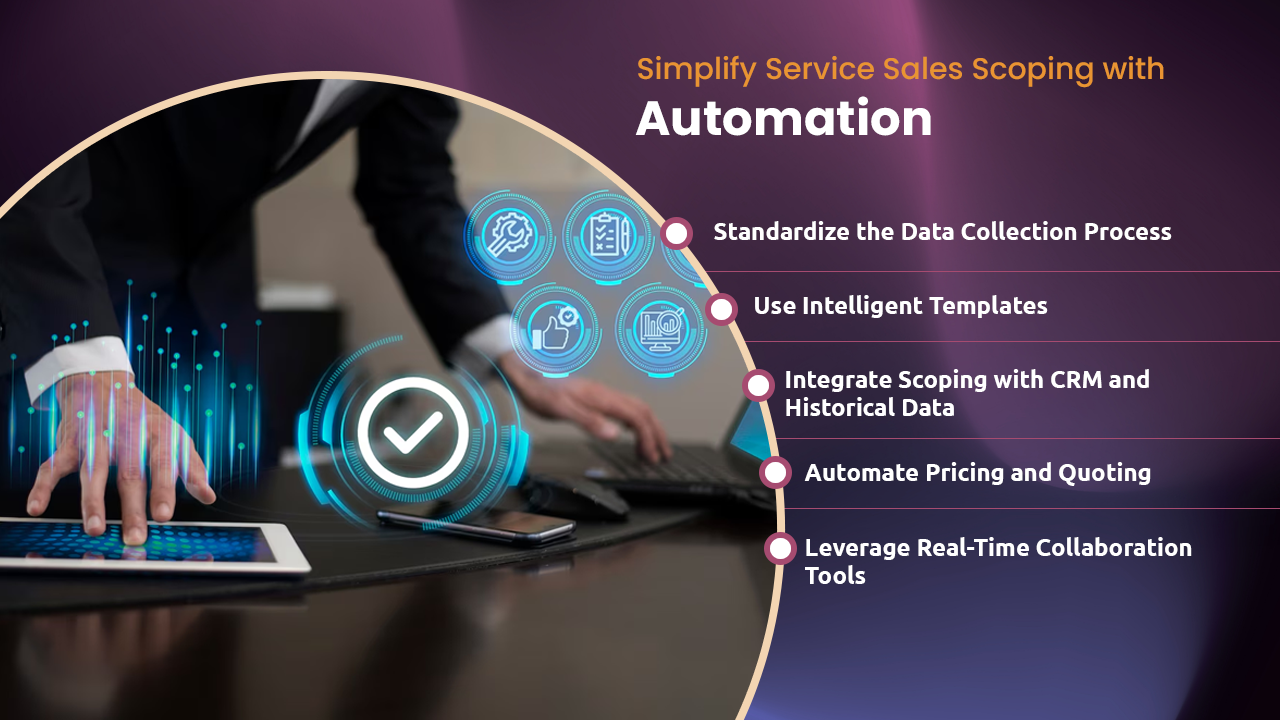
5 Ways to Simplify Service Sales Scoping With Automation
March 2025 | 69 min read
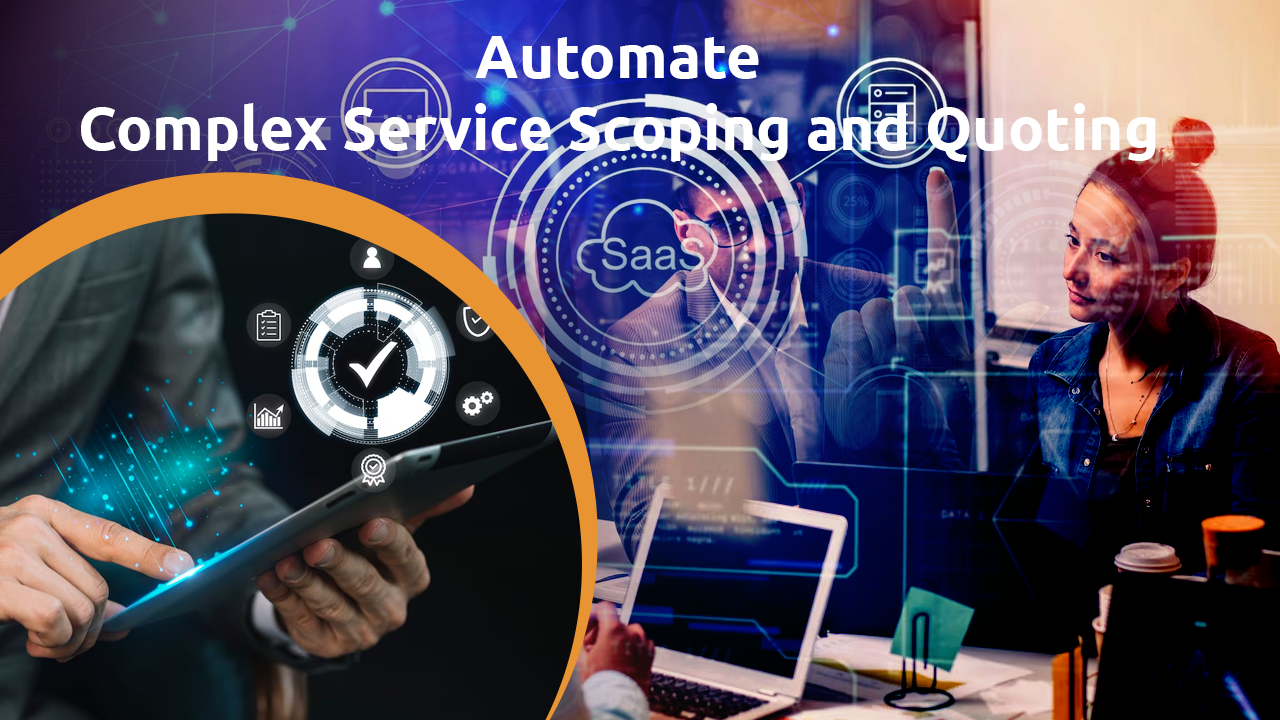
How to Automate Complex Service Scoping and Quoting for Professional Sales Teams
March 2025 | 111 min read
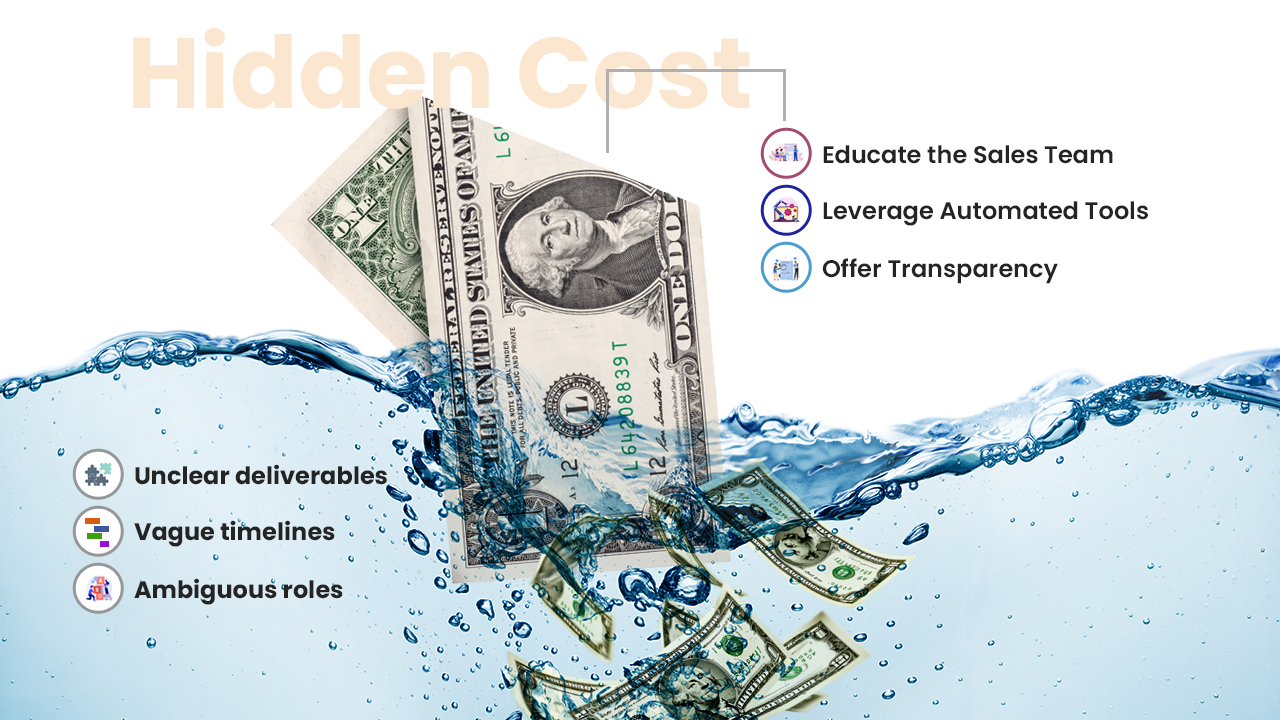
The Hidden Cost of Inaccurate SOWs in Service Sales
March 2025 | 66 min read